Expected goals, or xG, has become an increasingly popular metric in football analysis over the past several years. In simple terms, xG aims to quantify the quality of scoring chances in a match by assigning a probability value to each shot that reflects how likely it is to result in a goal. But how exactly is xG calculated, and why has this statistic grown in prominence? More importantly for bettors, how can expected goals data be leveraged to gain an edge in football betting markets? This article will provide an in-depth look at expected goals, its variations, and practical examples of how it can enrich match analysis and provide valuable insights for bettors like you.
Expected Goals Definition
Expected goals is a statistical measure that calculates the probability of any given shot resulting in a goal, based on a huge dataset of hundreds of thousands of historical shots. Each shot’s xG value is calculated by feeding key variables like shot location, angle, assist type, and defensive pressure into a complex model trained on this historical data. The output is a number between 0 and 1 representing that shot’s likelihood of being scored.
A shot taken close to goal in a central position with no defenders nearby may get an xG value of 0.6, meaning we’d expect it to result in a goal 60% of the time. A speculative long-range effort or tightly marked shot would have a much lower xG, such as 0.05. A penalty, one of the biggest chances existing in football, has an xG value of 0.76 and is fixed. However, other shots can have slightly different xG values depending on the data provider that calculated the shot as each of them will have their own unique model and data they train the models on. The xG values of all shots in a match are aggregated to generate expected goals totals for each team. This measure then serves as an insight into how many chances a team created and what quality these chances had.
How Are Expected Goals Calculated?
There are several models for calculating expected goals, with Opta’s being one of the most sophisticated. Their model considers over 20 match variables for each shot, including:
- Distance and angle to goal
- Type of shot (header, volley etc.)
- Body part used (left foot, right foot)
- Number of defenders between shooter and goal
- Goalkeeper position and likely reaction time
- Quality of pass received and type of build-up play
By factoring in so many influential variables, Opta’s model can estimate the likelihood of each shot resulting in a goal with a high degree of accuracy. Their database contains nearly 1 million historical shots from which the model has “learned” the importance of each factor in determining shooting success.
Other models may use fewer variables but still produce fairly reliable expected goals estimates. In general, the more contextual data included, the more accurate the xG model will be.
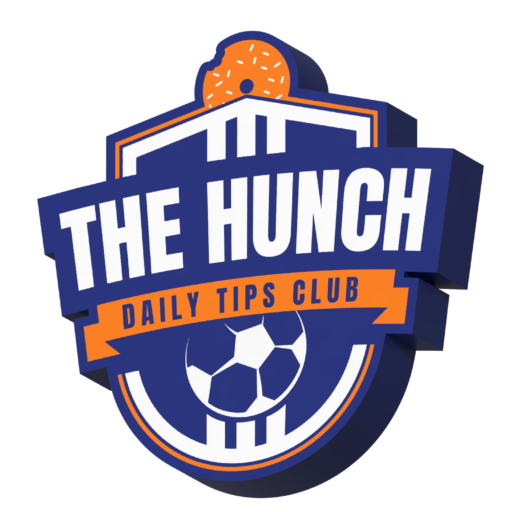
At the Hunch club we use expected goals data as one of the many variables in our models, for more info about our free community click here!
Variations of Expected Goals
There are several variations of expected goals that provide more granular insights:
- Expected Goals per 90 (xG/90): The average xG value per 90 minutes for an individual player. Allows comparison of players’ goal threat while normalising for playing time.
- Non-Penalty Expected Goals (npxG): Expected goals minus penalties, which have a fixed conversion rate. Gives a clearer view of chance creation from open play.
- Expected Goals For (xGF): The total xG for shots a team takes over a given period. Measures attacking threat.
- Expected Goals Against (xGA): The total xG conceded by a team over a given period. Assesses defensive performance.
- Expected Assists (xA): The xG value of chances a player creates through passes, indicating chance creation ability.
- Expected Points (xPts): The number of points a team is expected to earn over a
period based on xG ratios in their matches. Evaluates overall performance.
All these metrics are great assets to help you analyse previous matches and distil team and player performance. This knowledge will prove extremely valuable in sports betting.
Why Has Expected Goals Been Introduced?
Expected goals has risen in popularity because it provides richer insights into match events and patterns than conventional stats like shots and possession. With the advancement of technology and data science capabilities we are increasingly able to collect,transform and use data in football and sports in general. Teams hire data analysts to help them prepare for upcoming matches whereas even mainstream media use data such as expected goals in their match analysis to inform the fans of underlying dynamics. Here are some of the key benefits xG provides for football fans and bettors around the world.
- More accurate match analysis: xG differentials better indicate which team created better chances and deserved to win.
- Assess team performance: xG For and Against reveal attacking threat and defensive solidity over time.
- Evaluate player contributions: Comparing xG to goals scored assesses finishing ability. xA measures creativity.
- Identify luck vs. quality: Teams/players overperforming xG may be lucky, while underperformers are wasteful.
- Predictive capabilities: xG correlates strongly with future match outcomes and league position.
In short, expected goals gives analysts and fans a deeper understanding of the game by quantifying chance quality in fine detail. It has opened up new avenues for data-driven analysis.
Remarkable Expected Goals Examples
Here are some memorable examples of teams and players over performing or
underperforming their expected goals to give you an insight of the use of Expected Goals in practice.
- Liverpool 7.21 – 0.36 Newcastle (April 2021): Liverpool massively exceeded their xG in this win, on the one hand this could be attributed due to wastefulness from forwards such as Darwin Nunez but also highlights the excellent performance of Newcastle goalkeeper Martin Dubravka.
- Man City 99 goals from 91 xG (2021/22): City’s consistency in converting chances helped drive their title win. They outperformed their xG by almost 10% which has been instrumental in securing their league title with 1 point over Liverpool.
- Harry Kane 18 goals from 19.57 xG (2021/22): Kane was unusually wasteful, scoring far fewer than expected. This has actually been the first and only season that Harry Kane scored fewer goals than his expected goals, highlighting his incredible talent as a striker. All the more evident at FC Bayern.
- David De Gea, Manchester United (2017-2018 Premier League Season):De Gea’s exceptional shot-stopping ability was evident in the 2017-2018 season. De Gea was crucial in Manchester United finishing in 2nd place as he conceded 10 goals fewer than would be expected.
- Leicester City’s Title-Winning Season (2015-2016):Leicester City’s entire season was marked by a substantial discrepancy between their actual points and the expected points based on their performances. If the league table would have been made up based on expected points, The Foxes would have finished in 4th.
These examples demonstrate how xG can reveal when teams/players are lucky or excelling versus struggling based on the quality of chances. These stats can help you in gaining deeper insights into match and team dynamics.
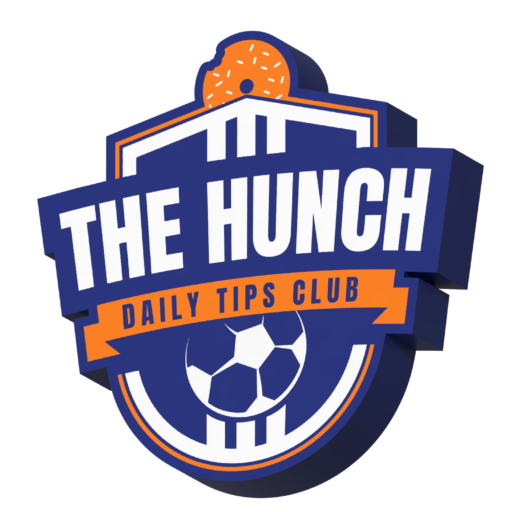
Expected Goals can be very useful metric to delve deeper into match statistics, read on to know how to use it as a bettor
Using Expected Goals In Football Betting
Expected goals data, when used properly, can give you a valuable edge by providing insights that purely looking at regular match stats cannot provide you. Knowing the underlying form and true quality of teams and players will help you tremendously in predicting future matches and aid you in finding the best bets with the right odds available on the market.
Here are some of the key ways it can inform betting decisions:
- Evaluate team performance: xG metrics can reveal which teams are creating/conceding higher quality chances than their results indicate.
- Identify runs of form: xG over time shows if a team is performing well but unlucky or riding an unsustainable streak of luck.
- Assess player goal threat: Comparing xG to goals can find players likely to score more or less than their current output suggests.
- Estimate match outcomes: xG line bets offer value when the oddsmaker’s goal projections differ from xG estimates.
- Model team strengths: xG differential and PDO (save percentage + shooting percentage) can improve predictive models for match outcomes.
However, data should always be just one input into your analysis, supplemented by other key factors like injuries, tactical changes, and qualitative assessments of team performance. The oddsmakers also utilise xG in pricing markets, so simply targeting teams that exceed their xG may offer limited value.
Limitations of relying on Expected Goals In Football Betting
While insightful, expected goals are not a magical potion that will magically help you predict every single football match and make you a millionaire sports bettor in 6 months. There are definitely various limitations to what xG can and cannot help you achieve. Taking these limitations into account when crafting your betting strategy is instrumental as a savvy bettor.
Some of the common limitations include:
- Past performance: xG is based on past statistical patterns and doesn’t account for future changes in personnel, tactics, or psychology and is thus only limitedly of use in predicting future matches.
- Data availability: Bookies also use expected goals data in their models, so their pricing more likely than not is reflected in the odds. This makes finding a consistent edge more difficult, even when using expected goals in your system.
- Sample size variance: xG predictions can be skewed by small sample sizes and outlier events that may not sustain. In a single match expected goals can be heavily overreached or under reached. Even when measured over an entire season there can be gaps between expected goals and actual goals.
- Qualitative factors: Team morale, injuries, and tactics can all influence future performance beyond what pure xG data suggests.
- Individual Player Anomalies: A striker can consistently outperform their xG throughout a season, regularly scoring goals from difficult or unlikely positions. This could be attributed to the player’s exceptional finishing skills, ability to capitalise on defensive errors, or a unique style of play that the xG model might not fully capture
- Underestimating Defensive Prowess: A team with a historically strong defence could consistently concede fewer goals than their xG against would suggest. This could be due to factors such as an exceptional goalkeeper, effective defensive organisation, or a tendency to limit opponents to low-quality scoring opportunities.
The best approach is to incorporate xG as one input among many, rather than relying on it as a predictive silver bullet. Used judiciously, it can certainly help refine projections, but a holistic analysis is still required to find value in football betting markets.
At the Hunch Club for example, expected goals for both teams in match data are one of the over 30 variables we take into account into our machine learning models. And even with these variables we still make sure that we use a sensible staking and bankroll management strategy to optimise our results. If you want to know more about our approach, join our free community where we provide you with daily tips and insights into betting.
Conclusion
Expected goals is an insightful statistic that provides a more sophisticated measurement of chance quality than conventional figures like shots and possession. Factoring historical shot data into probabilistic models, xG offers quantifiable estimates of chance likelihood that enrich match analysis and player/team evaluation.
Expected goals data offers valuable information often overlooked by the market. Comparing xG to actual goals scored can reveal teams and players likely to improve or regress in terms of scoring rates. Used carefully in conjunction with other key analytics, xG can help uncover potential betting value. However, its limitations as a predictive tool based on past data must be acknowledged.
By simultaneously knowing the power but also the limitations of metrics like expected goals you can access new levels of insight to get an edge. But the oddsmakers also have extensive data science capabilities. Beating them requires an intelligent, selective approach that blends quantitative and qualitative analysis. If applied sensibly, expected goals can form one part of a successful betting process.
Recent Videos
-
Become a Better Bettor
What are Expected Goals and how to use it in betting